This post actually just exists to make a point.
Framing Lenses – My Defence
Monday, November 10, 2014, I have successfully defended my master’s thesis and can now call myself M.Sc.
That is great and of course I want to share this with the world. So, my full thesis can be found here and it’s a great read, but I do understand if that is too much for you. So alternatively, you can just look at the presentation or decide to read further on when there is the text of my defence. Note that there has been no adjusting of punctuation, since the text was supposed to be spoken, not read.
Welcome to my Master’s defence, the final step of the Computer Science and Media programme.
Here, we will discuss my thesis, which has been my main occupation this year. As you can see, I have been investigating questions about the Framing of Gameplay Experience in Games with Eye Movement Based Adaptation.With this work I tried to find out, whether there is a placebo effect for games that are presented with a novel technological feature.
There are a lot of questions that could be asked now, and during the time of this presentation, I will try and address some of them.`
First, I will give a short introduction of the relevant terms and explain why it could be interesting at all. Then I will show the conceptual design of the adapted Tetris game and the conceptual design of the study as well as selected results together with their interpretation. Finally, I will present some options for further research.
But let’s start with the most obvious question: What do the words of the title even mean?
Consider framing.
While the nature of framing is studied in sociology, I use the broad definition from psychology, where framing refers to the presentation of information with different, but equivalent labels.
Concretely, what I did can be seen as attribute framing. While I did not present something positively or negatively, I did highlight different attributes (and omit others).This lead to the first part of my research question: If we suggest technological novelty, how does that fare to actually implementing it? (We will see the answer later.)
First we need to establish, what is meant by Gameplay Experience.
There are tons of expressions and words that are thrown around within academic discourse to refer to gameplay experience or aspects thereof. Some appear often: presence, involvement, flow and immersion. I will not explain them in detail right now, you can find out more with the given references, but all in all, there is a quality to playing games and digital games, that has no canonised term in academia yet. This makes it extremely difficult to measure this quality, since it is not even really defined yet.
However, I was not set out to solve this problem, so I relied on a standardised questionnaire, the Game Experience Questionnaire, because it was the best, available for my purposes and also covers most of the previously shown aspects. All other questionnaires referred to either narrative structures or overly visual experiences, which are both not given in Tetris, the game, I was looking at.
This leads to the second part of the research question: If we suggest technological novelty, how does Gameplay Experience change compared to games actually implementing it?
To continue with the inquiry about the long title, we have to look at what eye movements might tell us.
In general, eye movements can be distinguished in two large classes. Fixations and saccades. Fixations are the relatively stable episodes in which actual visual inspection happens. One of them lasts about 250 ms. Saccades are the very fast eye movements between fixations lasting about 55ms.
Eye movement analysis can also happen on a more finegrained and derived level, but they are all based on fixations and saccades. It is important to know that previous research indicates that experts have overall less fixations and larger saccadic amplitudes, this means, their saccades cover larger areas before a new fixation occurs. Relevant for the adaption processes I will show you in a few slides, are also focus areas, which accumulate fixations in an area of interest. Experts tend to have more fixations in these areas.
For Tetris this is the contour and the current tetromino.
There are also transitions, which describe how often the point of interest has changed between areas on the screen.
Here, there is an indication, that a user is more uncertain if there are frequent changes of focus. Look at the areas here and the fixations how they start here and then change in another area and stay there a little.
And there are scanpaths, which are an illustration of the direction of focus change over time. Especially for Tetris, Underwood illustrated in 2005, that experts show more horizontal than vertical eye movements in Tetris. In order to record these eye movements, eye tracking is usually done with a high frequency camera pointed at the eyes which is either located under the area of inspection or mounted on the head of the person who’s eyes are being tracked. I have used a head mounted version, the EyeLink II by SR Research, because it was the one with the highest temporal resolution available to me at the time.
With this data about eye movements, we can further elaborate on the research question. If we suggest technological novelty, how do Gameplay Experience and Expert Behaviour as measured by eye movements change compared to games actually implementing it?
In fact, I had a total of18 hypotheses which were again divided in different parts and all driven by different parts of the research question, but the main research question comes down to this,
where technological novelty is an aspect of games with eye movement based adaptation.
So let me rephrase: What has more effect on Gameplay Experience and expert behaviour as measured by eye movements: The implementation of eye movement based adaptation or simply the suggestion of this technology?
I want to explain now, why this is at all an interesting question for me.
So far I’ve only shown you a glimpse of relevant previous research and due to time constraints I am also not able to delve any deeper. There has also been our previous study on Hex, where we asked players whether they would have acted differently if informed beforehand that their eye movements are used. Most of them agreed. In the informed control group, however, more people stated that they didn’t act or behave differently. The data we had collected in this case was non-conclusive, so the question whether there is a palpable effect became more and more interesting to me.
Additionally, I am trying to do science with a critical approach I learned via the other field I studied; media culture. This means, I try to ask novel questions driven by a critical stance towards technological development. So, looking at this cool new thing we developed – in our case eye movement based adaptation – I’m trying to find out whether it makes sense on its own and how much of an effect can be attributed to the simple novelty of the technology. It appears that this question is rarely asked in sciences with a mainly positivistic view on its outcomes.
Furthermore, with the availability of comparatively cheap eye trackers with good enough frame rates and an option to grab data in real time, the development of games using those eye movements has a new momentum. Just last month the CHI Play conference had its own workshop on Applications for Gaze in Games (called EyePlay). Most of the projects conceptualised there use eye movements as an input device. While I have the feeling that this might be a bit frustrating, when imprecise measures (how they still occur even in eye trackers with well adjusted head tracking), when these imprecise measures lead to unintended inputs. However, taking more derived measures to adapt to eye movements in a stepwise or even historic fashion seems to be more promising to me as single eye movements only contribute partly to an accumulated effect.
There are several ways to accomplish this, let me show you my version of eye movement based adaptation.
I have used Tetris in my research, because it is visual and also spatial. Next to the obvious modifier of difficulty — the speed in which tetrominoes, the Tetris pieces, appear, the random choosing algorithm that decides which piece comes next can be altered. I did a small pre-study with 16 participants in order to find out, which algorithms are more difficult than others. It turns out, that a lot relies on the frequency of s and z pieces occurring – the zigzaggy ones noone appears to like anyway. With this knowledge I had a second way to adapt Tetris.
In order to have a control version, that is adaptive but not using eye movements, the fully adaptive process uses components with and without eye movements jointly. This makes it possible to later check whether there is an effect of eye movement based adaptation that cannot be attributed to adaptation in general. To adapt to algorithms, I have assigned numbers to them. The non-eye movement based adaptation mode is bumpiness. It describes the variance in height differences along the columns of a current game. The transition value tells us about the confidence with which a player is playing. This means, the more transitions over time, the less secure a player is. High bumpiness as well as a high transition value lead to an easier algorithm. However, the rationale here is that even if a player plays comparatively well, if they feel insecure about their performance, the difficulty should be adjusted differently.
For speed adaptation, I chose pile height. Remember the original Tetris game, pile height is the measure by which speed is adjusted. Every time, the pile height is reduced, the speed is increased. In my version, as soon as a player reached a pile height of five for the first time, I decreased the speed a little bit. The increase still happened faster than the decrease in order to not completely counteract a player’s expectations in how the game works. Then the eye movement based speed adaptation checked how often players looked in areas of special interest. This means the more focused players were, the faster the speed.
In order to see, what the effect of eye movement adaptation really is compared to its suggestion, I needed four groups. Players who were framed as in told that their eye movements were used for adaptation had to play games with eye movement based adaptation and games without. Players who were not framed needed to play both games as well. Since in the pilot study players were not able to distinguish the different types of adaptation, I was able to divide the real test persons in groups according to framing and letting both groups play both types of games. This means, of my 43 test participants 22 were informed about the use of eye movements whereas 21 were not informed. Each participant played 2 games with eye movement based adaptation and 2 games without. During all games their eyes were tracked, which was important for the results, but also to not give an external indication about which type of adaptation was used.
So, what were my results?
This graph shows means and the error bars depict one standard deviation from that mean. This is the case for all of the following figures as well.
First of all, I could show that the games are significantly different when eye movements are used in the adaptation process. The average value of the algorithm is higher in games with Eye Movement Based Adaptation, leading to a harder game. Harder algorithms produce less fitting pieces; hence, it is harder to make lines.
The speed was also significantly different between the two modes of adaptation. Conventionally adapted games ended on a higher speed than games that also used eye movement based adaptation. This means, that the pressure during the game came more from the speed at which the pieces appeared and fell down instead of ill-fitting pieces in conventionally adapted games, whereas in eye movement based games, the difficulty came from the shapes more than from the speed at which the pieces fell down. All in all it can be established that the adaptation via transition values indicating uncertainty of players overall added to the difficulty since it was solely targeted at the piece choosing algorithms whereas the analysis of expert fixations lead to an overall easier game. Seeing as the performance did not differ significantly across the modes of adaptation, it was interesting to me to check whether there has been a different experiential quality to the games.
In conventionally adapted games, framed players reported a significant difference in experienced challenge. Note, just the framing was different. This difference in experience is also not reproduced for eye movement based games, which indicates that the expectations of framed players shaped their perceived challenge. This does also indicate that games with eye movement based adaptation are expected to be more difficult.
It is furthermore interesting to see that framed players also perceived their competence differently according to which game they were facing. In games with eye movement based adaptation they reported significantly more competence than in conventionally adapted games. This means, that when their expectations were actually met, as in they expected a game to use their eye movements and it actually did, they felt like their strategies were having a bigger effect, which cannot be established by the actual performance measures.
Finally, there is also a significant difference in expert related eye movements at least in conventionally adapted games. This measure has not been analysed for games with eye movement based adaptation, since there for those that is an input variable. So, in conventionally adapted games, framed players show significantly less fixations outside of the scope of the interesting regions than non-framed players. This indicates that framed players were more concentrated on relevant points of the game.
Thinking back at the research question. Originally I was set out to determine what has more of an influence on players: The novel technique of eye movement based adaptation or its simple suggestion.
Taken these findings together, it turns out that eye movement based adaptation as well as framing have different effects on players. One without the other is less influential than both together; but personally, I would consider it unethical to only have one anyway.
What can we now do with these findings?
Well, first there are things that could be done better. Not only is it probably more desirable to use a remote eye tracker instead of mounting a heavy piece of hardware on a players head, but, it it also important to incorporate qualitative methods in a similar study. I have no way of telling you about the story where I had brother and sister in my lab playing at different skill levels but being equally impressed by each other’s performance and having similar levels of fun; I cannot tell you this story in a structured fashion because I had no methods recording this reliably and my quantitative measures don’t suffice to describe experiences adequately. Also the expectations framed players had are not recorded although they have often been verbalised before the game or even during.
Finally, there is also further research that can be conducted from here. Other games could be interesting, especially more complex and dynamic ones. Hex and Tetris rely heavily on turns and episodes, maybe a fluid game produces different eye movements. Also the development of eye movements over several episodes could be interesting. This could also lead to a prediction of likely eye movements in certain situations and with an algorithm anticipating those, eye movements could be lead whenever that is suitable (e.g. by making areas pulse in order to draw attention to them). It might also be fruitful to consider different patterns of eye movements. For example people with autism look at different areas and different details in an image. If the presented visual structure of a game is more complex than Tetris, this might play a role for gameplay experience.
However, I think I have been able to contribute to our knowledge in two core ways: I have done an investigation into framing effects of the suggestion of technological advance and with this I have done critically informed quantitative research. Furthermore and in order to do so, I have conducted an extensive user study of which I have shown you an overview of the results right now. I hope, this is a contribution that adds to science beyond the fact, that I personally might achieve an academic degree from it.
That was it from me for now. Thank you for your attention. Are there any further questions?
Space Antenna Hat
So I did the first Space Invader Hat, that I made a dozen pieces of and everybody was happy. But there is always someone, who want something different. In order to fulfil all needs brought towards me, I made this second hat. And I want to share the pattern with you folks. It is very similar to the original; only the middle part is changed. However, I give you the full list of steps again, so you don’t have to switch between references.
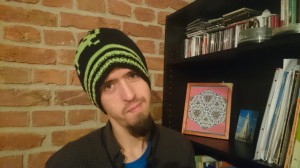
Materials you need:
- 3.5 mm double pointed needles (dpns) (or circulars for magic loop method)
- 2 balls of yarn (I used Lana Grossa Cool Wool for that project, but any sports weight will do) in different colours (I will use the actual colours used here to explain the pattern). You will only use up about half of the inserted colour (here: green).
- stitch markers
- tapestry needle
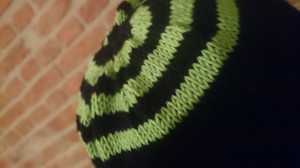
Abbreviations (a.k.a. stitches you should know):
- k – knit
- p – purl
- ktbl – knit through back loops
- k2tog – knit two together
- stb – switch to black
- stg – switch to green
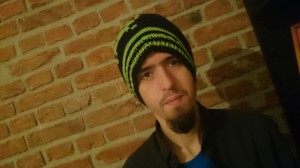
Techniques you should know (because I will not explain them; there are already so many helpful videos and tutorials out there!):
- intarsia
- intarsia in the round
- how to switch colours effectively horizontally and vertically
Pattern Note:
- 2×2 rib: 2ktbl, 2p (throughout the round)
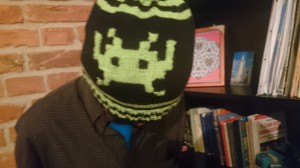
Steps:
- with black: cast on 120 stitches
- distribute stitches on needles (if you use dpns) as you like, join the round, being careful not to twist
- work 3 rows in 2×2 rib
- stg, repeat step 3
- stb, repeat step 3
- repeat step 4
- stb, k 3 rows
- stg, k 3 rows
- repeat step 7, k 3 more rows
- k48, stg, k3, stb, k18, stg, k3, stb, k48 (you start intarsia in the round for the end of the round)
- p48, stg, p3, stb, p18, stg, p3, stb, p48
- repeat step 10
- p51, stg, p3, stb, p12, stg, p3, stb, p51
- k51, stg, k3, stb, k12, stg, k3, stb, k51
- repeat step 13
- k51, stg, k18, stb, k51
- p51, stg, p18, stb, p51
- repeat step 16
- p48, stg, p24, stb, p48
- k48, stg, k24, stb, k48
- repeat step 19
- k45, stg, k9, stb, k3, stg, k6, stb, k3, stg, k9, stb, k45
- p45, stg, p9, stb, p3, stg, p6, stb, p3, stg, p9, stb, p45
- repeat step 22
- p45, stg, p3, stb, p3, stg, p18, stb, p3, stg, p3, stb, p45
- k45, stg, k3, stb, k3, stg, k18, stb, k3, stg, k3, stb, k45
- repeat step 25
- k45, stg, k3, stb, k6, stg, k3, stb, k6, stg, k3, stb, k6, stg, k3, stb, k45
- p45, stg, p3, stb, p6, stg, p3, stb, p6, stg, p3, stb, p6, stg, p3, stb, p45
- repeat step 28
- p51, stg, p3, stb, p12, stg, p3, stb, p51
- k51, stg, k3, stb, k12, stg, k3, stb, k51
- repeat step 31
- k 6 rows
- stg, k 3 rows
- stb, k 3 rows
- stg, (k13, k2tog) around, k 1 row, (k12, k2tog) around
- stb, (k11, k2tog) around, k 1 row, (k10, k2tog) around
- stg, (k9, k2tog) around, k1 row, (k3, k2tog) around
- stb, (k2, k2tog) around, k1 row, (k1, k2tog) around
- thread yarn twice through remaining stitches and pull tightly
- weave in ends
- set your intarsia straight
- block if wanted
- done!
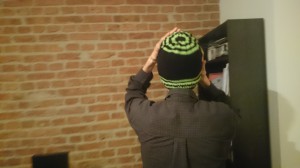
Hextris
After I did research on a game of Hex and also now doing research on Tetris, I am very very happy, to present to you now the gem my partner found online: Hextris. It’s Tetris on a Hexagon. It blew my mind.
Space Invader Hat
Years ago, I started these and gave them all around. However, I never published a pattern for them. With an upcoming move, I remembered that I promised someone to make them one and haven’t managed in five years (there are _good_ reasons for that, though). Finally, I made another one and this time, I thought of writing things down. So enjoy this little friend. (I also plan on doing one with another space invader, so if you want a more iconic version, stay tuned.)
Materials you need:
- 3.5 mm double pointed needles (dpns) (or circulars for magic loop method)
- 2 balls of yarn (I used Lana Grossa Cool Wool for that project, but any sports weight will do) in different colours (I will use the actual colours used here to explain the pattern)
- stitch markers
- tapestry needle
Abbreviations (a.k.a. stitches you should know):
- k – knit
- p – purl
- ktbl – knit through back loops
- k2tog – knit two together
- stb – switch to black
- stg – switch to green
Techniques you should know (because I will not explain them; there are already so many helpful videos and tutorials out there!):
- intarsia
- intarsia in the round
- how to switch colours effectively horizontally and vertically
Pattern:
- 2×2 rib: 2ktbl, 2p (throughout the round)
Steps:
- with black: cast on 120 stitches
- distribute stitches on needles (if you use dpns) as you like, join the round, being careful not to twist
- work 3 rows in 2×2 rib
- stg, repeat step 3
- stb, repeat step 3
- repeat step 4
- stb, k 3 rows
- stg, k 3 rows
- repeat step 7, k 3 more rows
- k48, stg, k3, stb, k3, stg, k3, stb, k6, stg, k3, stb, k3, stg, k3, stb, k48 (you start intarsia in the round for the end of the round)
- p48, stg, p3, stb, p3, stg, p3, stb, p6, stg, p3, stb, p3, stg, p3, stb, p48
- repeat step 10
- p51, stg, p3, stb, p3, stg, p6, stb, p3, stg, p3, stb, p51
- k51, stg, k3, stb, k3, stg, k6, stb, k3, stg, k3, stb, k51
- repeat step 13
- k54, stg, k3, stb, k6, stg, k3, stb, k54
- p54, stg, p3, stb, p6, stg, p3, stb, p54
- repeat step 16
- p48, stg, p24, stb, p48
- k48, stg, k24, stb, k48
- repeat step 19
- k48, stg, k6, stb, k3, stg, k6, stb, k3, stg, k6, stb k48
- p48, stg, p6, stb, p3, stg, p6, stb, p3, stg, p6, stb, p48
- repeat step 22
- p51, stg, p18, stb, p51
- k51, stg, k18, stb, k51
- repeat step 25
- k54, stg, k12, stb, k54
- p54, stg, p12, stb, p54
- repeat step 28
- p57, stg, p6, stb, p57
- k57, stg, k6, stb, k57
- repeat step 31
- k 6 rows
- stg, k 3 rows
- stb, k 3 rows
- stg, (k13, k2tog) around, k 1 row, (k12, k2tog) around
- stb, (k11, k2tog) around, k 1 row, (k10, k2tog) around
- stg, (k9, k2tog) around, k1 row, (k3, k2tog) around
- stb, (k2, k2tog) around, k1 row, (k1, k2tog) around
- thread yarn twice through remaining stitches and pull tightly
- weave in ends
- set your intarsia straight
- block if wanted
- done!
Sunflower Felting Pins – Pattern
For the last election campaign, we needed small tokens to identify our candidates again. This time I made felted pins in the shape of sunflowers. And now that campaigning time is over, I want to share the pattern.
Materials needed
- Felting Mat
- Scissors
- Fine Felting Needle
- Sewing Needle
- Some Thread
- Empty Pin
- Yellow and Green Felt
- Green Felting Wool
Steps
1. Cut out a small circle-ish shape from the green felt.
2. Cut out leaves (as many as you want/need) and start felting them on individually.
3. In order to create a nice shape, start with four you distribute roughly around the circle. You will felt the leaves on on the back.
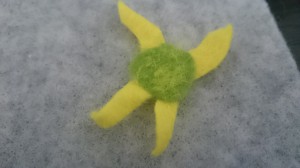
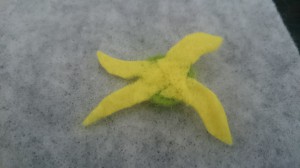
Then you add up on pedals as much as you want. Keep on adding them from behind and creating a sturdy back. Here is an intermediate view front and back.
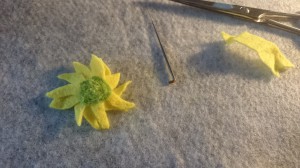
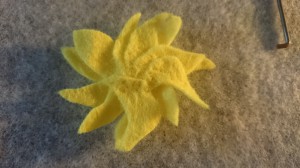
When you’re satisfied with your flower, sew the empty pin on.
Finally, take the little bit of loose felting wool and felt it loosely into the middle.
And done! Just attach it wherever you want and carry the sun around with you.
<3
Pytris – A Python Script for Testing Tetris Algorithms
In another post, I explained a probably non exhaustive list of the different types of Choosing Blocks Algorithms that might exist for Tetris. Here I explain Pytris, a script that has the logging abilities for research into the algorithms. This is part of my ongoing Master’s Thesis Project. If you’re interested in the source code, just contact me! I’m happy to provide it with additional explanation.
Pytris has been implemented in Python under heavy use of the pygame library. This made it possible to account for the desired goal of having test participants create a realistic game environment on their own computer. With pyg.exe even Windows users were enabled to play the game on their operating system.
Game Mechanics
Pytris offers all game mechanics, that can generally be found in any implementation of Tetris. Players are able to translate and rotate pieces coming down. Additionally, they can listen to the original Tetris score while playing, if they desire to do so.
Upon starting the script, Pytris captures the screen in a full-screen mode so that players are not visually distracted on the screens of their computers. Graphically there are also no further distractions from the game itself. It does not show a score or a number of lines made indicating performance directly.
The initial speed of the game is set to 400 ms, which means the tetromino is moved every 400 ms. Speed increases of 5 ms happen whenever a line is removed. If more than one line is removed at the same time, the speed increase is multiplied by the number of lines removed. The minimum speed is set to 75 ms. These parameters can be changed, if needed.
For each game the algorithm for choosing pieces is chosen at random without replacement from a bag holding all five basic algorithms described above, each of them twice. This means, each player plays ten games of Tetris, two per algorithm in a random order.
A game ends after five minutes or if a player looses – whichever event comes first. The script pauses upon that point in order to give players time to fill in a questionnaire or have a self paced break between games. However, during these breaks the screen is still captured preventing players to do any other computer based activity, at least at the machine they are playing Pytris on. Due to the time restrictions for each individual game, players play a maximum of 50 minutes during one session.
Data Recording
Pytris has been designed in order to record a lot of data in general, not only for the data analysis required for this research. For each game within a session, a log file is created. After a test session has been concluded, these files can be retrieved in a folder named by the participant ID.
Every entry in a log file has a timestamp. A log file consists foremost of a header for general test data such as the participant ID defined in the player setup, the number of the game (ranging from 1 to 10), which algorithm has been used in this game, whether the player had the original Tetris score activated and finally, when the game started. The initial speed and every speed change are recorded as well.
For each new block, a situational analysis is performed. This consists of a count of lines made so far into the game, the current pile height, the current bumpiness measure, the current number of closed holes and for which type of tetromino there are possible placements on the current contour. Finally, the chosen block and the current grid are recorded as well as every keyboard interaction of the player.
Different Tetris Algorithms
I am currently writing my Master’s thesis and using Tetris as a game of reference. In a pre-test I needed a quick version of Tetris, that records a lot of the interaction and tests different algorithms for choosing blocks. Before I present the resulting implementation (Pytris), I want to briefly talk about algorithms that choose blocks in Tetris. Part of this post is in the draft of my written thesis as well.
But lets get into the heart of Tetris: how to choose blocks! Next to obvious solutions like a random choosing of all available blocks, there have also been propositions of using Tetris games as means of communication by encoding information in how the blocks are chosen. This selection of blocks is then still supposed to appear random or at least semi-random to an unknowing player of such a game (see for an example Ou, 2011).
In this post, the five algorithms implemented are presented with the general idea behind how the blocks are chosen. An exemplary implementation of each algorithm is provided in Python.
Nicetris
In order to offer players an algorithm that helps them learn the game and play it with a minimum amount of challenge, the Nicetris algorithm has been designed specifically for this work, but with the inverted principles of the Bust Head (see below) algorithm. By analysing the situation of the current game board and especially, how the contour looks like.situation[4] in the code example refers to an array containing all shapes, that are fitting the current built-up. If the current built up is really not fitting for any piece (which is theoretically impossible), the choice is made from the set of generally well fitting blocks (O, I, and L-blocks).
nice_bag = []
for element in bag:
if element in situation[4]:
nice_bag.append(element)
if len(nice_bag) > 1:
return choice(nice_bag)()
else:
return choice([I,O,L])()
This approach was designed to ensure that the player always has an edge-fitting option to place the current tetromino and, hence, enable them to clear rows fast.
Grab Bag
According to the Tetris Wiki, this is the original Tetris algorithm. Essentially, all possible tetrominoes are put in a bag and drawn randomly one after the other without replacement, which means, until the bag is empty. Then, another bag is started for the next seven pieces.
if len(ungrabbed_bag) == 0:
ungrabbed_bag = [ O, I, S, Z, L, J, T ]
block = choice( ungrabbed_bag )
ungrabbed_bag.remove(block)
return block()
This is assumed to create a fair random game. The properties of this algorithm are, that there are at most 12 pieces between two I tetrominoes and a maximum of four ß pieces can come in a row. Hence, the chances of encountering a run of the same (bad) pieces are eliminated.
True Random
The most basic algorithm for choosing blocks in a game of Tetris is the True Random version.
return choice( O,I,L,J,T,S,Z )()
This algorithm is equal to drawing from an urn of tetrominoes with replacement. Very fortunate and very unfortunate series of blocks are likely in an equal way. However, this algorithm might actually sometimes show stronger patterns than the others.
Skewed Random
In order to increase the likelihood for a kill sequence as described by Burgiel, 1997, Skewed Random assigns a 50% chance to either of the ß (S or Z) pieces instead of the usual likelihood of 2/7 = 28.57%.
if random.randint(0,1) == 0:
return choice([S,Z])()
else:
return choice([O,I,L,J,T])()
Mild Skewed Random
A milder version of this algorithm adapts Skewed Random as such, that the likelihood for ß pieces are at about 39%. This version is expected to still be more difficult than the previously described algorithms (Nicetris, Grab Bag and True Random) while being less harsh than the 50% version.
Bust Head
This algorithm is inspired by the Bastet game developed by Frederico Poloni. While his version relies on a well analysis, the version used in this research is based on a contour analysis, but with a similar idea. First, the algorithm checks, which pieces do not fit the contour (fitting pieces are recorded in an array in situation[4] in the code example). Then, a bag of these pieces plus the O piece is used to randomly choose the next tetromino. If every possible tetromino could fit the contour, the undesirable combination of O and ß pieces is used as a bag for the next elements.
tiny_bag = [O]
for element in bag:
if element not in situation[4]:
tiny_bag.append(element)
if random.randint(0,2) > 0:
if len(tiny_bag) == 1:
return choice([O,S,Z])()
else:
return choice(tiny_bag)()
else:
return choice(bag)()
While Bastet and Bust Head share the same core idea, which is to create a really difficult game of Tetris, their methods of achievement differ. Hence, the algorithm used here is named differently, but phonetically similar in order to keep the roots of the idea in mind.
I hope this helped a bit in understanding that randomness can be achieved in different ways and how the choosing block algorithm in Tetris can be manipulated. This helps then in understanding the soon to come implementation description for Pytris.
Beach in Langballigau
Last week we spent some time at the Baltic Sea. There is a tragicomic story to tell about how I lost my phone and found it again in the beach two days later although the cover was done in a sandy colour. It still had 50% battery, alas I didn’t have it for the day at Legoland. Enjoy these for now.
[BMo_scrollGallery id=14 sG_thumbPosition=bottom sG_images=1 duration=slow gallery_width=600 gallery_height=400 thumbs_width=100 thumbs_height=100 sG_caption=1 sG_start=1 sG_loop=1 sG_loopThumbs=1 sG_clickable=1 sG_opacity=40 sG_area=200 sG_scrollSpeed=2 sG_autoScroll=0 sG_aS_stopOnOver=1 sG_diashowDelay=0 sG_followImages=1 sG_responsive=1 ]
Talk ‘Surveil and Calculate’ at XII Conference on Culture and Computer Science
In May I was at the XII Conference on Culture and Computer Science in Berlin and gave this talk when it was accepted as a contribution to the conference by a peer reviewed abstract. That was also my first conference ever at which I was talking, so I was a little nervous. However it worked out and I have even been asked to put this online for others to share and find, which I do here happily.